Researchers develop a tool that will enhance warfighter data sharing
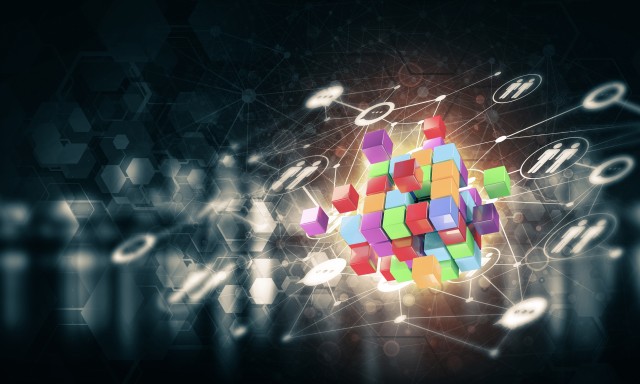
ADELPHI, Md. -- Army researchers developed a tool that will enhance warfighter data sharing across various platforms by innovatively addressing cyber, physical and cyber-physical threats, and making privacy-preserving data sharing easier than ever before.
The research, supported by the U.S. Army Combat Capabilities Development Command’s Army Research Laboratory’s Cybersecurity Collaborative Research Alliance, the National Science Foundation, Siemens, Google and JP Morgan Chase, also has the potential to help create realistic training testbeds for network security engineers without exposing sensitive real data.
According to researchers from Carnegie Mellon University and IBM, limited data access is a longstanding barrier to data-driven research and development in the networked systems community. In this work, they explore if and how generative adversarial networks, or GANs, can be used to incentivize data sharing in privacy-sensitive settings by enabling a generic framework for sharing synthetic datasets with minimal expert knowledge.
The researchers developed a tool called DoppelGANger, which models time series data and evaluates it on networking- and systems-relevant datasets. As a specific target, the focus of this research is on time series datasets with metadata.
“We identify key challenges of existing GAN approaches for such workloads with respect to fidelity, such as long-term dependencies, complex multidimensional relationships and mode collapse, and privacy, as existing guarantees are poorly understood and can sacrifice fidelity,” said Zinan Lin, CMU doctoral student.
With DoppelGANger, the researchers demonstrated that across diverse real-world datasets (e.g., bandwidth measurements, cluster requests, web sessions) and use cases (e.g., structural characterization, predictive modeling, algorithm comparison), DoppelGANger achieves up to 43% better fidelity than state-of-the-art baseline models.
When it comes to privacy, although the researchers do not resolve the privacy problem in this work, they identified fundamental challenges with both classical notions of privacy and recent advances to improve the privacy properties of GANs, and suggest a potential roadmap for addressing these challenges.
In addition to achieving state-of-the-art results in time series modeling, which is a task of independent interest in the machine learning community, Lin said this work is tackling a major pain point in industry and academia today.
“Lack of data sharing is the root cause of many problems in the network, systems and cybersecurity domains, where entities are reluctant to share data about security incidents due to fear of revealing sensitive data to competitors and/or violating user privacy,” Lin said. “There is a pressing need for tools that can make privacy-preserving data sharing easier. We have open-sourced DoppelGANger with detailed documentation so that everyone can use this workflow for data sharing.”
According to Lin, several companies have already used DoppelGANger on their own internal datasets and confirmed the fidelity of DoppelGANger.
Prior data synthesis approaches such as simulation and mathematical models usually require domain experts to configure complicated parameters of simulators, or to specify which statistics in data are important for modeling, Lin said. Those approaches cannot easily generalize across different datasets and use cases and require significant human efforts.
On the contrary, DoppelGANger requires little or no prior knowledge of the data because of the capability of GANs and therefore can generalize across different datasets and use cases with minimal parameter configurations.
“Even among GAN-based approaches for modeling time series, we achieve state-of-the-art performance by designing an architecture that explicitly encourages the generation of longer time series, diversity of samples and joint generation of metadata along with time series,” Lin said.
According to Professors Vyas Sekar and Giulia Fanti, CMU professors and Lin’s advisors, this work is most relevant to the modernization of Army network technologies.
DoppelGANger can be used to share data between divisions of an organization that are not able to directly share information due to privacy or security restrictions, they said.
Instead of waiting for network engineers to diagnose a problem and synthesize a summary of a sequence of events, DoppelGANger could be used to release a synthetic version of an observed malicious network traffic.
This architecture also enables operators to modify metadata distributions if these encode sensitive information.
Another possible future benefit according to the professors is that synthetic network data can be used to help create realistic training testbeds for network security engineers without exposing sensitive real data. The flexibility of DoppelGANger could help the creators of training exercises tune or adapt the generation of synthetic data to the desired application.
Sekar and Fanti also envision such tools helping in-field operations where data sharing may be necessary across a federation of different entities such as Army battalions from different allies working cooperatively and sharing intelligence to address cyber, physical and cyber-physical threats.
“This research is highly relevant to the Network Army Modernization Priority, specifically through the use of advanced machine learning techniques towards cyber and cybersecurity information domains,” said CCDC ARL researcher Dr. Kevin Chan. “In general, there is a lack of availability of training data for machine learning-based network applications. This research has strong potential to support future Army operations by expanding the capabilities and settings to which machine learning can be applied. Given that machine learning is data hungry, labeled network data is not readily available, so this gives us tools to fill this gap.”
For Sekar and Fanti, they are optimistic that the proposed tools are a step towards circumventing the practical and political barriers that make data sharing difficult today.
“We believe that future organizations will need to flexibly utilize all available data to be able to react to an increasingly data-driven and automated attack landscape,” Sekar said. “In that sense, any tools that can facilitate data sharing are going to be essential. We are hopeful that these tools can enable the Army of the future to design more flexible and streamlined internal tooling for sharing information about ongoing network events.”
Moving forward, the researchers will explore various avenues for this tool to expand its capabilities even further.
“Many networking datasets require significantly more complexity than DoppelGANger is currently able to handle, such as causal interactions between stateful agents,” Lin said. “Another direction of interest is to enable “what-if” analysis in which practitioners can model changes in the underlying system and generate associated data.”
Although DoppelGANger makes some what-if analysis easy, Lin said, larger changes may alter the physical system model such that the conditional distributions learned by DoppelGANger are invalid (e.g., imagine simulating a high-traffic regime with a model trained only on low-traffic-regime data). Such what-if analysis is likely to require physical system modeling/simulation, while GANs may be able to help model individual agent behavior.
The privacy insights developed in this work also highlight the need for improving the fidelity-privacy trade-offs of current privacy training techniques, he said.
In addition, the techniques proposed inside DoppelGANger for dealing with long time series and jointly generation of metadata and time series are not only applicable for networking/systems datasets, but can also potentially helpful for many other types of data like text, speech, music, financial, and medical data. The researchers are currently evaluating DoppelGANger on such domains, particularly in the finance sector.
“The overall objective of the Cyber Security CRA is to develop a fundamental understanding of cyber phenomena so that fundamental laws, theories and theoretically grounded and empirically validated models can be applied to a broad range of Army domains, applications and environments,” said Dr. Michael Frame, collaborative alliance manager. “This alliance brings together government, industry and academia to develop and advance the state of the art of cyber security, and this collaborative effort shows the success of working together and combining research and experiences all in support of our warfighters. We look forward to continuing and expanding upon this research to further change how Soldiers communicate safely and securely on the battlefield.”
This research, recently presented virtually at the ACM Internet Measurement Conference 2020, a three-day event focusing on internet measurement and analysis, earned recognition as Best Paper Finalist.
Visit the laboratory's Media Center to discover more Army science and technology stories
CCDC Army Research Laboratory is an element of the U.S. Army Combat Capabilities Development Command. As the Army’s corporate research laboratory, ARL is operationalizing science to achieve transformational overmatch. Through collaboration across the command’s core technical competencies, CCDC leads in the discovery, development and delivery of the technology-based capabilities required to make Soldiers more successful at winning the nation’s wars and come home safely. CCDC is a major subordinate command of the Army Futures Command.
Social Sharing