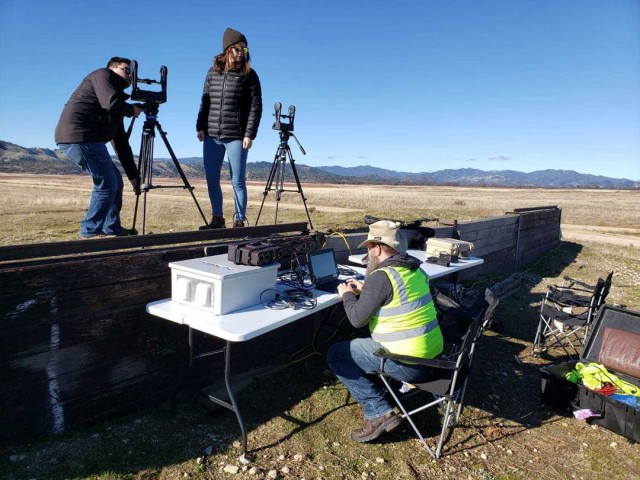
Our world creates, collects and is driven by data. Google, Facebook, your insurance company and even your local grocery store collect data and use it to better their chances for success. When done correctly, that advantage leads to increased profits. We need to do the same thing: create and collect our acquisition data and explore how we can apply it through artificial intelligence (AI) for acquisition success.
Why artificial intelligence? Because we need help in the complex world of systems acquisition and because we lack the right tools to bring developments in on time and on budget. We are always behind. The tools and processes developed in the 20th century analog world are just not effective in the digital world of the 21st century. We need a new tool to bring programs in on time and on budget. Artificial intelligence, backed by big acquisition data, is that new tool. We must start thinking about how AI can help program managers (PMs) today. And that starts with the acquisition experts defining what we want AI to do.
IDENTIFYING THE PM AND ACQUISITION TASKS FOR AI
Today, weapons systems development is a people-intensive, hands-on activity requiring paperwork, bureaucratic processes and communication. In this age of COVID-19, a work-quarantined PM friend recently told me that he is finally getting used to not having paper in his hands to read and make decisions. He quite proudly told me, “I am finally getting used to looking at the reports on a screen rather than having them put in my inbox every day.” While he is a bit of a dinosaur, the fact is many of us are not wholly on board with everything digital. We need to get that way.
The second thing he said struck me as well. “I never realized the drudge work of project management.” He was used to moving around and travelling, and staying at home forced him to focus on the not-so-fun parts of his job. It’s too bad it took him this long, but most of us already know acquisition isn’t all drama and excitement. It has tasks that require the human touch, but the fact is, project management can be rudimentary, repetitious and in many cases, just boring. We need to get smart and let machines do the boring work.
Stephen Wolfram, a prominent scientist and software executive and CEO of Wolfram Research, describes the challenge of AI. It’s not the ability to perform high-level tasks—because it can. Instead, he says, the challenging issue is humans being able to describe in detail exactly what we want AI to do, because AI out-of-the-box has no value. In order to describe exactly what we want AI to do, we need to take a look at what we do in acquisition. From there, we can decide.
What is it that AI should do? We get there by asking what it is that the PM and staff do. People in DOD, warfighter and PM alike, know what needs to be done, and what they alone must do that cannot be delegated to a computer. Once we have figured out that division of labor, we can think about what goes into AI.
A good place to start is with the PM. PMs solve problems and coordinate program activities. They also plan for what’s next and wargame strategies to address upcoming events, whether milestone reviews or testing. Lastly, PMs must spend time communicating with their people formally through reviews and counseling.
While these are broad areas, each offers opportunities to shift effort to those things that allow a PM to influence the activities, rather than being a “rider on the bus.” For example, planning an acquisition program is a major effort. We start with a blank whiteboard and spend significant time developing a plan. We can identify previous, similar programs, whether aircraft or tank, and use AI to provide a plan outline and milestones. Further, if we have collected the data, we can examine planning assumptions and their actual outcomes against our proposed plan. Finally, AI can compare our cost and schedule estimates against historical actuals.
Another example, risk management, is a major part of control. While every program is different, the causes, likelihood and consequences of risk have some degree of similarity. AI, with the appropriate historical data and training, can help a PM anticipate risk occurrences and often provide the time needed to prevent a risk from turning into a showstopper. Automating risk warnings are a way to reconfigure the PM’s time.
We must examine all of our processes, from contracting to systems engineering, from planning to program control, to decide where AI can best help us do our jobs better.
Think of AI as an expert consultant that you hire to come into your program. Consultants bring knowledge and an outside perspective informed by their understanding of best practices across an industry. They track the lessons learned. They are experts focused on the details necessary for success. They can bring their data and lessons learned into your program. That captures the essence of what AI can do. Decide what you want and define the consultant-like capability that can reside within your program, available 24/7 to inform, and if necessary, provide you advice.
CARE AND FEEDING OF AI
We have data. Lots of it. We have to ask why we collect what we do. Much of the data we gather today is a result of legal and regulatory requirements. We have to define how it should be formatted. Machines can only work with specific formats. And the data has to be organized, accurate (no mistakes or typos), complete (no missing fields) and, most importantly, in a format that can be translated into AI through machine learning.
We also must get serious about the data we need, related to what we want AI to do. We are great in cost data because that is easy to understand and measure, and we have taken the time to develop meaningful tools.
We need to get better in schedule and performance data by explaining better what causes changes from the plan, and what we can do to get back on the plan. For instance, there are a finite number of reasons a schedule changes. Capturing those reasons for use in AI can be a powerful planning tool to allow future PMs insight into potential schedule problems. Finally, we need to capture our thinking in both planning and execution. In planning, we should be articulating our assumptions and, while executing, describing whether those assumptions were valid or not and, more importantly, why.

Getting to AI
We get to AI through machine learning. The data provides historical information and lessons learned. Machine learning is an iterative and cyclical process that depends on user and system feedback. Users must provide comments on the fielded system capabilities and problems to allow constant improvement through regular updates, which will also incorporate newly generated data.
AI identifies patterns in the data to match those to something occurring in a program’s execution today. It does this through machine learning, the enabling process of making data into AI. Machine learning allows computers to act or provide information without direction from an operator. One of the ways it does this is through sorting large amounts of data, referred to as “big data,” to identify those patterns.
Warfighters use this pattern recognition capability to identify changes in enemy activities—changes that only appear on examination of large amounts of data over long periods of time. Physicians use pattern recognition to assist in identifying cancer in X-rays. In acquisition management, we are looking for patterns of current activity that match something that has happened elsewhere, on another program, at a different time.
Pattern matching isn’t the only capability we can expect from AI, though. Statistics are also key to machine learning and AI. Statistics offer the possibility of prediction using simple regression techniques to more sophisticated mathematical modeling. Prediction helps in planning and estimation, as well as risk tracking and mitigation. Prediction also provides a longer-range perspective (with the right data) by constantly comparing the present status using all available data with that of past, similar developments.
System development is a human activity that requires humans to do things in a particular way. We must examine critically whether the processes, both those based on best practices and those based on bureaucratic requirements, contribute to the mission and make sense. More importantly, we must be sure they are efficient. AI can and will do what we teach it, but it can’t think and it can’t tell if something makes sense.
Connecting the Dots
There is good news in the potential for AI to address the complexities of weapon system development, but AI can’t fix everything. AI offers the possibility of radically changing the PM’s role from reactive to proactive. The biggest challenge for PMs is to clearly define their expectations for AI so the system will contribute to program success.
The biggest challenge for DOD is the data—whether it’s the right data, in the right quantities and if it is clean and accurate. To perform at an optimal level, AI requires big data and the data must relevant to the way we do business.
Data collected “because we’ve always done it that way” wastes valuable effort that could be better focused on getting exactly what we need. That requires a focus on the data collection and reporting that we don’t have today.
As a NATO-ally friend of mine once told me, “You Americans are world class at collecting lessons learned. However, I don’t see you really learning any lessons from the data that you collect.”
Artificial intelligence offers the possibility of using the history captured in acquisition data, the lessons learned, to enable PMs to address the complexity of weapon system development head-on and learn from our lessons. AI can’t replace PMs, but its potential offers the chance for the defense acquisition community to get ahead of the relentless complexity of system development and be able to get cost, schedule and performance right. And, it can permit us to get ahead of the problems for once.
CHARLES K. PICKAR is a senior lecturer at the Naval Postgraduate School (NPS) in Monterey, California, where he teaches program management, acquisition and systems engineering in the Graduate School of Defense Management. He has more than 30 years of management and research experience, including leadership positions at the Johns Hopkins University Applied Physics Laboratory, Science Applications International Corp., and Lockheed Martin Corp. He holds a doctorate in business administration from Nova Southeastern University, an M.S. in systems engineering from The Johns Hopkins University, an M.A. in national security affairs from NPS and a B.A. in business from the University of Maryland. He is a graduate of the U.S. Army School of Advanced Military Studies. The author’s last Been There, Done That column was “An Exercise to Experience,” in the fall 2019 issue.
Read the full article in the Summer 2020 issue of Army AL&T magazine.
Subscribe to Army AL&T - the premiere source of Army acquisition news and information.
Social Sharing