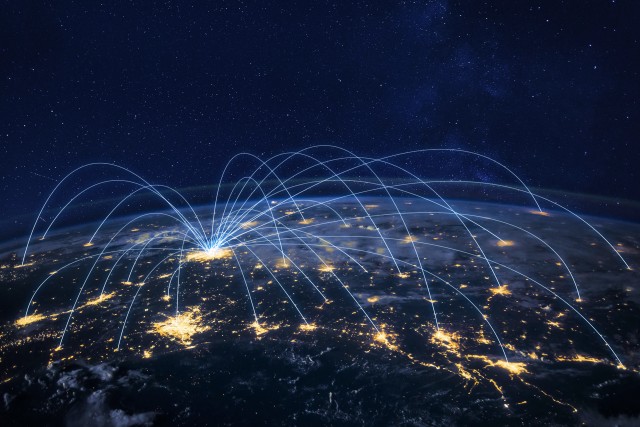
ADELPHI, Md. -- Army researchers developed a novel algorithm to protect networks by allowing for the detection of adversarial actions that can be missed by current analytical methods.
The main idea of this research is to build a higher-order network to look for subtle changes in a stream of data that could point to suspicious activity.
Most analytics build up first order networks, where edges represent a movement between two nodes. For instance, airports connected by direct flights. The history of multi-hop travel by people is lost in such networks. Higher-order networks include additional nodes to also represent the dominant (multi-hop) flows in the data.
The research focuses on harvesting social signals to detect emerging phenomena by looking beyond first-order Markov patterns over network data.
The work developed a representation that embeds higher-order dependencies into the network such that it reflects real-world phenomena and scales for big data and existing network analysis tools. It uses the representation to perform network analytics to identify influential nodes, detect anomalies and predict co-evolution of multi-genre networks.
“We developed a scalable and parameter-free algorithm for higher-order network representation, BuildHON+, building on our prior work,” said Dr. Lance Kaplan, researcher with the U.S. Army Combat Capabilities Development Command’s Army Research Laboratory. “We demonstrate the efficiency of BuildHON+ through comprehensive complexity and performance analysis on global ship movement data, which is known to exhibit dependencies beyond the fifth order, meaning, for example, we predict the next port based upon more than the past five ports that the shipment has passed through.”
This work is the result of a collaboration under the laboratory’s now concluded Network Science Collaborative Technology Alliance between Kaplan, Mandana Saebi, Jian Xu, and Nitesh Chawla from the University of Notre Dame, and Bruno Ribeiro from Purdue University. They were able to showcase the performance of BuildHON+ in the task of network-based anomaly detection on both real-world and synthetic taxi trajectory datasets.
To do this, the collaborators created a synthetic dataset of origins and destinations for taxi cabs. In the real world data set, there was only one abnormal day that could be detected. The synthetic data set enabled a more systematic comparison of the BuildHON+ against first order network approaches.
“Using a large-scale synthetic taxi movement data with 11 billion taxi movements, we show how multiple existing anomaly detection methods that depend on first-order network collectively fail to capture anomalous navigation behaviors beyond first-order, and how BuildHON+ can solve the problem,” Kaplan said.
According to Kaplan, most analysis of streams over network data assume first-order Markov evolution, i.e., the probability that a ship or taxi visits a port/location depends solely on its current location in the network. The ability to represent higher-order dependencies enables one to distinguish more subtle traffic patterns.
The higher-order network representation results in a more accurate representation of the underlying trends and patterns in the behavior of a complex system, and is the correct way of constructing the network to not miss any important dependencies or signals, he said. This is especially relevant when the data is noisy and has sequential dependencies within indirect pathways.
Another way to describe this method is to look at shipment traffic.
“Consider ships traveling from port to port,” Kaplan said. “Each port is a node in the network. A first order network is where an edge between ports B and A represents the non-zero probability of a shipment from port B to port A. Higher order networks consider edges where the shipment at port B has already traveled through specific ports in specific order. The algorithm uses the data stream to build such higher order networks by using specialized statistical tests to progressively determine which next higher order edge is necessary or not to explore.”
By building up higher-order networks from the data streams at adjacent time intervals, he said, one can detect subtle changes in the data streams that traditional first-order networks would miss.
For instance, consider a small port E where all of a sudden there is a relatively large shipment of goods from port E to port D to port C to port B to Port A, but because port E is small, and most packages from port E go to port D anyway, the changes in the data stream would not change the structure of the first order network at all. However, Kaplan said, the higher order network method can potentially detect such changes.
In this example, the subtle change was because of a shipment of explosives to be used by a peer adversary in a region of conflict serviced by port A.
“This shows how subtle changes in a data stream of some supply/logistical network can provide intelligence of potentially nefarious activities,” Kaplan said.
This research has numerous applications, ranging from information flow to human interaction activity on a website to transportation to invasive species management to drug and human tracking, Kaplan said. For Soldiers, it could be applied to a supply/chain network used both by Soldiers and Civilians within an area of interest.
“The higher-order network analysis can find weak signals in a logistics network of adversarial actions that would be missed by first-order network representations,” Kaplan said. “This can include preparations by non-state actors to launch an attack in support of a peer adversary.”
Moving forward with this research, there are still a number of scientific questions that the team, and the scientific community at large, will continue to pursue.
“The concept of higher order networks opens up many different interesting avenues of investigation within network science to better predict the coevolution of networks and detect weak signals of adversarial behaviors,” Kaplan said.
For instance, he said, a potential research direction would be to generalize the notion of nodes into other network elements such as subgraphs or motifs so that one can better understand how social norms within the general population can evolve.
A second extension is to explore higher order networks in multi-layer networks representing different social groups or different modes of communication to increase the contextual fidelity to find weak anomalous signals. A related question is how to make the analysis robust to deception, where the streaming network data might be manipulated at a subset of the nodes.
Researchers said further testing and exploration will mature this technology for future Soldiers, keeping them safer and more prepared for the missions that lie ahead.
CCDC Army Research Laboratory is an element of the U.S. Army Combat Capabilities Development Command. As the Army’s corporate research laboratory, ARL discovers, innovates and transitions science and technology to ensure dominant strategic land power. Through collaboration across the command’s core technical competencies, CCDC leads in the discovery, development and delivery of the technology-based capabilities required to make Soldiers more lethal to win the nation’s wars and come home safely. CCDC is a major subordinate command of the U.S. Army Futures Command.
Social Sharing