ADELPHI, Md. — Army scientists developed an innovative method for evaluating the learned behavior of black-box Multi-Agent Reinforcement Learning, known as MARL, agents performing a pursuit-evasion task that provides a baseline for the development of adaptive multi-agent systems, known as MAS.
This state-of-the-art research is detailed in the paper, "Emergent behaviors in multi-agent target acquisition", which is featured in the SPIE Digital Library. The researchers said that this work addressed the Army modernization priority to develop Artificial Intelligence, known as AI, systems that can flexibly adapt to the human Soldier and the surrounding environment.
The researchers said that mission success would depend on sufficient coordination between AI systems and the Soldier enabling AI systems with critical action selection capabilities to provide the Soldier with improved safety, enhanced situational awareness, and general force multiplication. Researchers expect that such AI systems will be able to adapt to specific learning experiences to entirely new situations by adhering to the military operations Observe, Orient, Decide, and Act (OODA) loop.
Dr. Piyush K. Sharma, Army researcher and AI coordinator of the U.S. Army Test and Evaluation Command, is one of the scientists who contributed to the research, which he conducted previously while working as a computer scientist at the U.S. Army Combat Capabilities Development Command, Army Research Laboratory.
“It is expected that human Soldiers augmented with AI systems will have increased lethality and survivability in the future battles with technologically advanced adversaries”, said Sharma. “The pursuit-evasion game requires knowledge of the evader's (prey/target) location. The game is aligned with an Army Target Acquisition task, and this task is part of the larger intelligence, surveillance, target acquisition, and reconnaissance mission objectives.
According to Sharma, these objectives can be represented in scenarios with simplified environments to gain an understanding of how MAS might perform when trained with Reinforcement Learning, known as RL, approaches in the future battlefields of the U.S. Army's Multi-Domain operations .
The researchers utilized an MAS using RL in a continuous bounded 2D simulation environment to train and evaluate agents in a pursuit-evasion (a.k.a. predator- prey pursuit) game that shared task goals to target acquisition with the Multi-Agent Deep Deterministic Policy Gradient, known as MADDPG, algorithm. The researchers utilized the OpenAI Gym multi-agent particle environment repository for simulation environment.
Researchers created different adversarial scenarios by replacing RL-trained pursuers’ policies with two distinct (non-RL) analytical strategies, namely Chaser and Interceptor, and categorized an RL trained evader's behavior. Specifically, they used a pursuit-evasion game to emulate an abstracted target acquisition task to demonstrate how RL agent policies allow the emergence of different behaviors under varying adversarial conditions. See the figure below illustrating the chaser and interceptor behavior in the pursuit-evasion game.
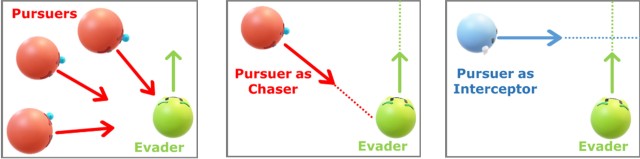
“MARL algorithms, such as MADDPG, have demonstrated the existence of coordination between teams of agents, indicating that they can produce emergent collaborative behaviors”, said Sharma. “If agents can identify these strategies, they can become more adaptive to new teammates by adjusting their behavior to accommodate their partner's strategy.”
Sharma explained how he and his fellow researchers have designed a method to identify the strategy employed by an agent within a pursuit-evasion game to work towards adaptable computational teammates.
“Our method is capable of meaningfully describing differences in team strategies that we verified by testing it on teams of agents with well-defined strategies”, said Sharma. “Specifically, our method can categorize differences in RL-trained agent (evader) behavior using a spatial histogram-based approach, which divides the simulation environment into bins (with different granularities/quantities for comparisons) and uses the bins as features for behavior classification.”
Sharma said categorization of differences in RL-trained agent (evader) behavior may aid in catching the (enemy) targets by enabling Sharma and other researchers to identify and predict enemy behaviors.
“When extended to pursuers, this approach towards identifying teammates' behavior may allow agents to coordinate more effectively,” said Sharma.
Unlike other mainstream AI research, this work was specifically designed to work within the battlefield settings.
“This work provides evidence that an agent’s RL trained policy may contain emergent behaviors that cannot be demonstrated unless the environmental conditions (e.g., adversarial behaviors) are different from the training environment,” said Sharma.
The researchers said they are optimistic this research work will lay the groundwork for future attempts to classify agent behaviors or team strategy. Their findings provide a foundational framework for future investigation aimed at deciphering the emergent behaviors from MARL agents.
“Leveraging what we learned during this research, we are better equipped to investigate particular aspects of MARL based approaches which train agents in centralized fashion,” said Sharma. “Future work will allow us to assess cooperative as well as adversarial coordination in the Army context.”
According to Sharma, despite the success stories in military operations, autonomous systems are far from being perfect and need to be appropriately evaluated before they can be deployed to defend critical territories.
“One example is Israel's Iron Dome defense system, which is claimed to have a success rate of 85% - 90% in intercepting approaching missiles within a 2.5 - 43-mile range” said Sharma. “For systems like this to work successfully, detection and tracking radars need to coordinate with a firing system, and an interceptor must be able to maneuver quickly. Even with these prerequisites in place, this success rate may not be acceptable when a missile is targeting critical infrastructure like a bridge or supply route. Therefore, our dependency on an inadequately tuned AI algorithm may result in poor performance with an unacceptably high false negative rate, leading to mission failure.”.
Moving forward, researchers feel this work is a step towards building an autonomous MAS by putting focus on training simulated agents in highly simplified and controlled environments to understand basic principles of multi-agent coordination. Researchers plan to move towards a real Army environment with the actual agents to further validate their approach and prepare for future battlefields.
Social Sharing