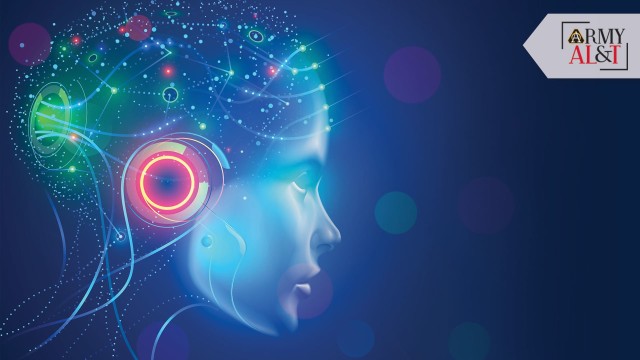
Tell someone you’re working on artificial intelligence for the Department of Defense, and there’s one cultural reference point they’re likely to mention—the “Terminator” franchise. As the story goes (and despite all of the movies in the series, it never gets particularly specific), robots advance sufficiently to gain consciousness and attack their human creators. “Killer robots?” they’ll ask, as if that’s exactly what you just claimed you do for a living.
It’s easy to get excited about AI because we now encounter it on a daily basis. Amazon has sold more than 100 million Alexa-enabled devices. We share the road with at least a few self-driving cars, and many more now park themselves. Netflix, Inc,. has more than 150 million subscribers, the result, in part, of the attraction of outside content, but Netflix increasingly produces its own content and matches subscribers to it via its recommendation engine. AI sure looks like magic, and is often referred to as such, with equal parts admiration and skepticism, as in the commonly heard phrase, “we’ll sprinkle some AI magic on this.”
Working with the nuts and bolts of defense data, though, it’s easy to see why Cyberdyne Systems, the developer of the AI entity in “Terminator,” would not have imagined that they were building toward something that would one day take over the world. Our data collection is inconsistent, storage is decentralized and standards vary from system to system. The Army’s recent data strategy addresses the importance of data to the military’s future and focuses on making data visible, accessible, understandable, trusted, interoperable and secure—though we’re still coming to grips with the implications of each of those factors.
Our systems development approach has long been based on the notion that we should express a desired capability and give industry maximum flexibility to identify solutions. This is the method we’re using now in the pursuit of “narrow AI”—an application that provides a well-defined, but limited capability. For example, software that determines when you’re likely to need resupply for a vehicle fleet based on user‑entered usage rates. Narrow AI is unlikely to surprise or delight us with ingenuity.
The need for a broader AI tool was highlighted by the Army National Guard’s efforts to support the national COVID-19 response. The question of how to ensure supplies got to a defined point of need was complicated by the spread of the virus, which reprioritized needs as new clusters emerged. Before we could align the supply chain for personal protective equipment or ventilators, we needed to know which hospital would require those supplies based on the spread of the virus.
Integrating, or fusing, data between two domains—for example, logistics and medicine—is a challenge, not only because those domains have specialized vocabularies, but also because they have different concepts. For fusion, we need a structure that can reconcile conceptual or semantic reasoning—both what something is, as well as how it relates to other things.
For the logistics community, a ventilator is a piece of equipment that is manufactured, stored, maintained and shipped. In the medical profession, a ventilator requires power, has a setting for oxygen level, is assigned to one patient at a time and may require certain medications for intubation. Knowing how to best get a machine from point A to point B is only part of the problem. A ventilator only represents a life saved if it arrives on time for a patient and the facility has the other elements required for successful use.
Data standards, that is, how data elements are defined and formatted, do not alone provide sufficient architecture for data fusion. For fusion, we need a structure that can reconcile conceptual or semantic reasoning in addition to allowing us to seamlessly send data between systems.
IT’S ALL ABOUT RELATIONSHIPS
The term “machine-understandable” harkens back to Skynet and killer robots—after all, if machines develop understanding, they must get how easy it would be to eliminate humans and take over the planet. Machine understanding, however, is more of a mechanical understanding than an existential one. An AI application may pass a Turing test, demonstrating its ability to behave in a convincingly human manner, but it will still lack other human traits, like appreciation of art or humor. While a standards-based data exchange like the National Information Exchange Model (NIEM), which the DOD nominally adopted in 2013, offers a framework for linking data elements across functional domains, it does not provide the structure necessary for a computer to move beyond representation of data and information to modeling knowledge or understanding. For that, we will need to adopt an ontology.
An ontology is a semantic model of data—that is, meaning is an emergent feature of how the data are related. In other words, it is a framework for applying shared meaning to data that humans and computers can understand. The building blocks of this model are “triples,” each containing a subject, a predicate and an object, which are understandable by both humans and computers. The subject and object are data elements and the predicate describes the relationship between them. These relationships can express a taxonomy, outlining that a brigade contains battalions and a battalion contains companies, and they can also define that a brigade is led by a colonel, or a squad contains between 4 and 10 Soldiers. This structure can be expanded to describe equipment and supplies, the capability of weapons, characteristics of maneuver and more.
Identifying the potential effect of isolated weapons on a particular target is a cumbersome task akin to delivery of a series of rocks—“Is this it?” Each weapon-target pairing is applied in series to determine the outcome. While this approach could be aided with tabulated look-up tables, deductive reasoning can provide solutions, often involving multiple weapons or tactics such as timing weapons in a series, to deliver a desired effect on a specified target. With a structure in place to provide the logic, an algorithm can determine which units have weapons with the desired effect on a selected target, as well as their ability to maneuver as necessary, given the speed of their vehicles and the state of their supplies.
IS IT DEDUCTIBLE?
Deductive reasoning is being applied to analysis of suspected chemical or biological laboratories. One could approach this problem by making an exhaustive list of materials and equipment used in the production of various substances and then comparing those lists to what is found in a given facility, but it’s difficult to be comprehensive.
A gas burner and a beaker might be the laboratory standard for heating a liquid, but if we look for those specific elements, we might miss the more common case of a heat source like a fire and a container capable of holding hot liquids like a metal pot. An ontology might specify that a pot has the capability of holding liquid and is composed of steel, while steel has the property of a high melting point, which allows heat to be applied to raise the temperature of a liquid contained in the pot.
This might seem intuitive to a human based on learned experience, but it is a fairly complex concept for a computer, though one apparently not lost to Arnold Schwarzenegger’s Model 101 terminator at the end of the second movie when he lowers himself into a vat of molten metal.
CONCLUSION
AI has long been a staple of science fiction, allowing mechanical beings to interact with humans on more or less equal terms. In the dystopian tradition, technology is often presented as a menace, like in the “Terminator” series, or HAL from “2001: A Space Odyssey,” but there are also more positive AI role models in popular culture, such as C-3PO from “Star Wars” or Tony Stark’s “Iron Man” interface, Just A Rather Very Intelligent System (JARVIS). One might imagine similar technology supporting our Soldiers, warning them of incoming threats and plotting opportunities through data-informed course of action analyses.
The Army Data Strategy includes a goal for making data understandable by users, but this should be expanded from human users to AI agents as well. Providing an ontology as a structure for machine understanding is essential for future AI applications. JARVIS embodies many of the capabilities the Army seeks to enable with AI, for example: automatic speech recognition combined with natural language processing, visual entity extraction and automatic target recognition, health monitoring and damage assessment. Absent a knowledge structure for machine reasoning, many of these capabilities cannot be realized.
Another goal of the Army Data Strategy is for data to be interoperable across systems. This is supported by data exchange standards, but adoption of NIEM and an ontology framework like Basic Formal Ontology (BFO) are not mutually exclusive. One can be used to supplement the other and both use universal resource indicators to unambiguously identify data elements. The development of conceptual domain ontologies is necessary for reasoning to span domains such as medicine and logistics, or fires and command and control, where the same data element may have different types of relationships. Because ontologies are extensible, allowing data elements to have different types of relationships, domains can be developed independently to an extent, but should still be governed by an umbrella framework such as BFO, to ensure those relationships are defined in a consistent manner.
The potential for computer reasoning to advance the Army’s ability to get supplies to hospitals at the time of need, or optimizing battle plans, represents a transformative future for our Army and our military.
“Killer robots?” they’ll ask.
“Maybe, but also robots that save lives.”
For more information, visit Project Manager (PM) for Mission Command, at https://peoc3t.army.mil/mc/.
THOM HAWKINS is a project officer with PM Mission Command, assigned to the Program Executive Office for Command, Control and Communications—Tactical, Aberdeen Proving Ground, Maryland. He holds an M.S. in library and information science from Drexel University and a B.A. in English from Washington College. He is Level III certified in program management and Level II certified in financial management, and is a member of the Army Acquisition Corps. He is an Army-certified Lean Six Sigma master black belt and holds Project Management Professional and Risk Management Professional credentials from the Project Management Institute.
KEN LORENTZEN is the chief engineer and technical management division chief for PM Mission Command. He holds a B.S. in engineering science (communication systems) from the City University of New York – College of Staten Island), an M.S. in software engineering from Monmouth University and an M.A. in management and leadership from Webster University. He is a member of the Army Acquisition Corps and certified Level III in both systems planning, research, development and engineering and program management, and is a graduate of the Defense Acquisition University Senior Service College Fellowship (2013). He is a veteran of the U.S. Navy and a graduate of Naval Nuclear Power School.
This article is published in the Summer 2020 issue of Army AL&T magazine.
Subscribe to Army AL&T – the premier online news source for the Army Acquisition Workforce.
Social Sharing